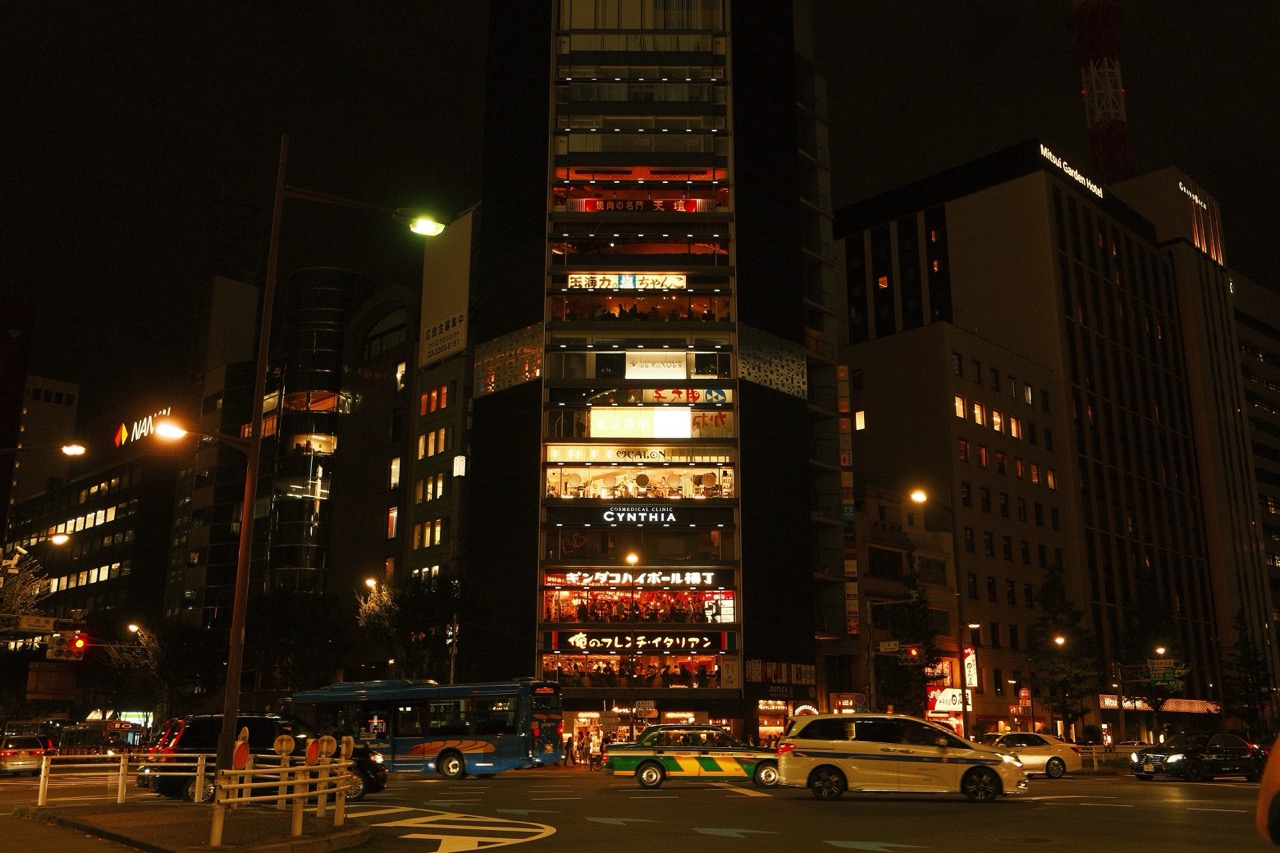
OWL-survey
@AikenHong2021 OWL 分析现有的OWL特点,和当前自己的研究做一个区分,也汲取一下别人的研究的要点, Reference arxiv @ self-supervised feature improve open-world learning arxiv @ open-world semi-supervised learning arxiv @ open-world learning without labels arxiv @ unseen class discovery in open-world classification arxiv @ Open-World Active Learning with Stacking Ensemble for Self-Driving Cars www @ open-world learning and application to product classification cvpr @ open world composition zero-shot learning cvpr @ Towards Open World Object Detection [cvpr](Large-Scale Long-Tailed Recognition in an Open World (thecvf.com) ) @ Large-Scale Long-Tailed Recognition in an Open World Conclusion Papers Mulit Open world Learning Definition ...